Master Thesis: Meta-Learning within the PAC-Bayesian Framework
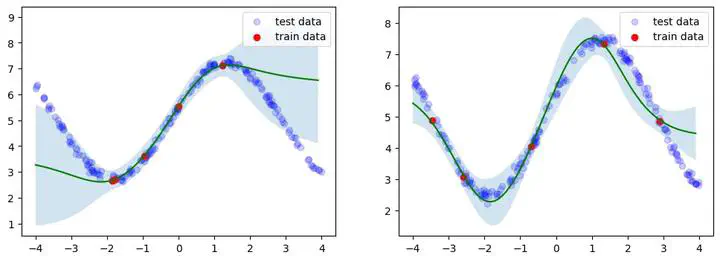
This master thesis delves into Meta-Learning, emphasizing PAC-Bayesian bounds for creating a theoretically grounded framework for meta-learners, notably the PACOH algorithm. It addresses the empirical nature of current Meta-Learning research, examining the PACOH as a solution with probabilistic performance guarantees. By exploring optimization-based meta-learners like MAML and REPTILE through a PAC-Bayesian lens, this work not only advances the theoretical underpinnings of Meta-Learning but also demonstrates the effectiveness of these approaches through numerical experiments. The thesis underscores the PAC-Bayesian Meta-Learning framework’s capability to contextualize and theoretically support existing algorithms, offering a robust foundation for future innovations in Meta-Learning.